What Is The Experimental Unit
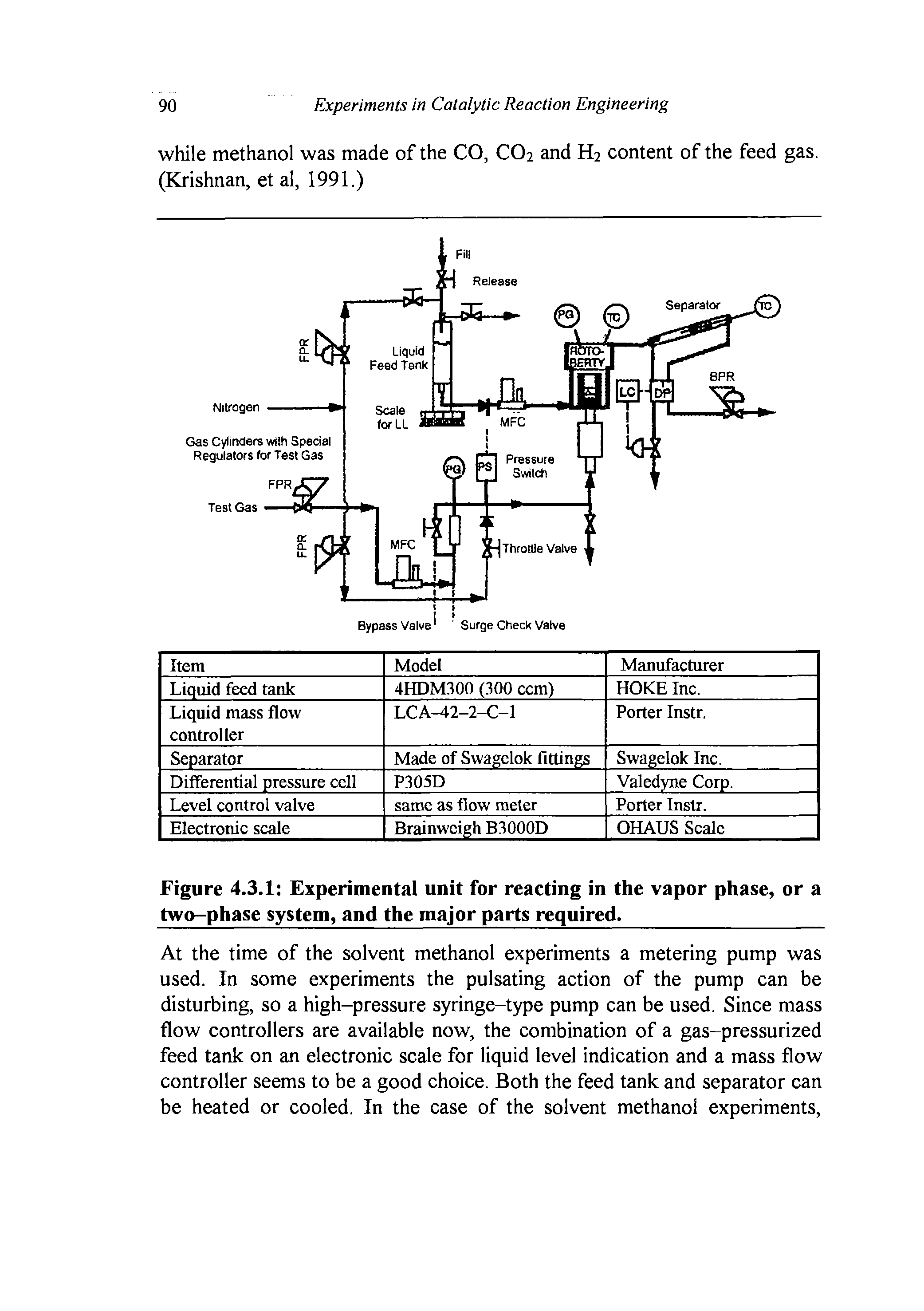
In the realm of experimental design and statistical analysis, the experimental unit is a fundamental concept that often goes unnoticed but plays a pivotal role in ensuring the validity and reliability of research findings. Whether you’re a seasoned researcher or a curious learner, understanding what constitutes an experimental unit is essential for designing robust experiments and interpreting results accurately. Let’s delve into this concept with clarity, examples, and practical insights.
What Is an Experimental Unit?
The experimental unit is the smallest entity to which a treatment or condition is applied and about which measurements are taken. It is the basic unit of analysis in an experiment. The experimental unit must be clearly defined to ensure that the treatment is properly assigned and that the data collected is meaningful.
Why Does the Experimental Unit Matter?
Misidentifying the experimental unit can lead to confounding or pseudoreplication, where the results appear significant due to artificial inflation of sample size rather than true treatment effects. For example, if multiple measurements are taken from a single experimental unit and treated as independent, it violates statistical assumptions, leading to incorrect conclusions.
Examples to Clarify the Concept
1. Agricultural Experiments
- Scenario: Testing the effect of fertilizer on crop yield.
- Experimental Unit: Each individual plot of land receiving a specific fertilizer treatment.
- Mistake to Avoid: Treating multiple plants within a single plot as separate experimental units (pseudoreplication).
2. Medical Trials
- Scenario: Evaluating the effectiveness of a new drug.
- Experimental Unit: Each patient randomly assigned to receive the drug or a placebo.
- Mistake to Avoid: Using data from multiple organs or tissues of the same patient as independent observations.
3. Educational Studies
- Scenario: Comparing teaching methods in classrooms.
- Experimental Unit: Each classroom assigned to a specific teaching method.
- Mistake to Avoid: Analyzing individual student scores without accounting for classroom clustering.
Experimental Unit vs. Observational Unit
It’s crucial to distinguish between the experimental unit and the observational unit:
- Experimental Unit: The unit to which treatments are applied (e.g., a field in agriculture, a patient in medicine).
- Observational Unit: The unit from which measurements are taken (e.g., individual plants, blood samples).
Aspect | Experimental Unit | Observational Unit |
---|---|---|
Role | Receives treatment | Provides measurements |
Example | Plot of land | Individual plant |

Common Pitfalls and How to Avoid Them
Practical Steps to Identify the Experimental Unit
- Define the Treatment: What intervention or condition is being applied?
- Identify the Entity: What is the smallest unit receiving the treatment?
- Verify Independence: Ensure each experimental unit is distinct and not influenced by others.
- Plan Data Collection: Align measurements with the experimental unit to avoid pseudoreplication.
Historical Context and Evolution
The concept of the experimental unit has evolved alongside the development of statistical methods. Early experiments often lacked clear definitions, leading to flawed conclusions. The work of statisticians like Ronald Fisher in the early 20th century emphasized the importance of proper experimental design, including the correct identification of experimental units.
Future Trends and Implications
As research becomes more complex, with interdisciplinary studies and big data, the precise identification of experimental units remains critical. Advances in statistical software now allow for better handling of hierarchical structures, but the onus remains on researchers to define units correctly.
FAQ Section
Can the experimental unit and observational unit be the same?
+Yes, in some cases, such as when measuring the effect of a drug on individual patients. However, they are often different, as in agricultural studies where plots are experimental units and plants are observational units.
What happens if the experimental unit is misidentified?
+Misidentification can lead to pseudoreplication, inflated Type I error rates, and incorrect conclusions about treatment effects.
How does the experimental unit relate to sample size?
+The number of experimental units determines the sample size. Treating subsamples as independent units artificially inflates the sample size, compromising statistical validity.
Can experimental units be nested?
+Yes, in hierarchical designs (e.g., students nested within classrooms). Proper statistical methods must account for this nesting to avoid bias.
Conclusion
The experimental unit is a cornerstone of sound experimental design and statistical analysis. By clearly defining and distinguishing it from the observational unit, researchers can avoid common pitfalls like pseudoreplication and ensure the reliability of their findings. As research methodologies evolve, the importance of this concept remains unchanged, serving as a critical tool for producing valid and reproducible science.